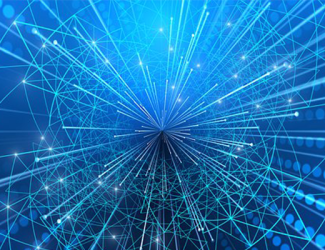
Torch.AI Announces New Data Transformations That Mobilize Messy Data and Provide Dynamic Enrichment to the World’s “Forgotten” Data Stores
November 16, 2020
Leawood, Kansas – November 16, 2020 – Torch.AI, creator of the AI/ML-powered Nexus™ Unifying Synaptic Mesh (USM) platform, today announced a feature release including Dynamic Data Transformations that will deliver value to customers by tapping into previously unapproachable data stores. The speed at which data is generated continues to increase, and overtime, much of that data remains dormant in unstructured formats, for example images, video, text-based, and audio files. Organization leaders struggle to integrate this data into their knowledge systems. This new feature announced today will enableTorch customers to work with more types of data at once, have more powerful and efficient data pipelines, and deliver structured, tagged, and searchable data to their diverse analytic workloads.
Data lakes and other cloud-based object storage facilitates the ease of saving an ever-increasing amount of unstructured data within an organization. Overtime, these unorganized storage containers are forgotten, or cast aside due to a lack of automation to make sense of them. Torch’s DynamicData Transformation is a prebuilt pipeline that can be configured to connect with hundreds of data sources or streams of data. As files are flowed into the platform, intelligent algorithms identify the file type—Microsoft Office documents of nearly any version, myriad imaging data types, PDF, audio formats—and queue files accordingly into their own channels for further deconstruction by ML algorithms into core data components. By automating the application of an organization’s taxonomy as metadata, every data asset withinTorch customers’ ecosystem is discoverable, cataloged, and prepped to power decisioning.
“Everyone has an S3 bucket, library, or data lake and they don’t even know what’s in it. They think, ‘Someday we’ll get to that,’” statesJon Kramer, Torch’s VP of Engineering & Development. “Customers say they only use 5% of the data they have because it’s so unstructured. Now Torch can tap into that data, ‘read’ it, deploy our NLP extraction techniques against the normalized content, and write tags against it.”
Torch envisions this feature enhancement will support customers in nearly every application, including person or facility vetting and whole customer understanding. The technology enables the collection of data artifacts filtered by entity. Using a person example, the result set may include images and video identifying the person using facial recognition, PDF documents referencing their name with NLP, or social media tagging their handle, scored by sentiment.
Copyright 2023 © Torch.AI
All rights reserved.
5250 W 116th Pl Suite 200
Leawood, KS 66211
1775 Tysons Boulevard
McLean, VA 22102